As Susanna Ray, in a blog post on the Microsoft website, explains, "The most advanced systems are showing the ability to go a step further, tackling increasingly complex problems by creating plans, devising a sequence of actions to reach an objective." And for us trying to understand the technical and legal aspects of AI and all it entails, Susanna gave a brilliant breakdown of some of the language that AI followers should become au fait with.
Language models are a critical component of AI systems that engage with human language. These models use machine learning techniques to recognize patterns and relationships in text data, allowing them to generate realistic and contextually appropriate responses. Small Language Models (SLMs), such as Phi-3, are compact and can be used offline on devices like laptops or smartphones. They are trained on smaller, curated datasets and have fewer parameters, making them ideal for handling basic queries or tasks.
On the other hand, Large Language Models (LLMs) are much more extensive and require significant computational power and memory. These models are capable of engaging in more complex reasoning and can generate more sophisticated responses. However, LLMs can sometimes struggle with separating fact from fiction or may rely on outdated information, leading to inaccurate outputs known as "hallucinations."
To address the issue of hallucinations and improve the accuracy and relevance of AI-generated content, developers employ techniques such as grounding and then subsequently Retrieval Augmented Generation (RAG).
Grounding involves connecting and anchoring the AI model with data and tangible examples to produce more contextually relevant and personalised outputs.
RAG enables the AI system to access additional knowledge sources without requiring retraining, saving time and resources.
As Ray explains, "It’s as if you’re Sherlock Holmes and you’ve read every book in the library but haven’t cracked the case yet, so you go up to the attic, unroll some ancient scrolls, and voilà — you find the missing piece of the puzzle."
AI systems often rely on an orchestration layer to manage the sequence of tasks required to generate the best possible response to a given input. This layer can store chat history, allowing the AI to understand the context of follow-up questions, or it can search the internet for up-to-date information to enhance the model's answers.
While AI models do not possess memory in the traditional sense, developers are experimenting with orchestration techniques that simulate short-term and long-term memory. This allows AI systems to temporarily store relevant information for the duration of a conversation or to retain important data for future use.
Transformer models, such as the one used in ChatGPT (Generative Pre-trained Transformer), have changed the field of AI by enabling systems to better understand context and nuance. They do so by paying attention to patterns in data and weighing the importance of different inputs.
In contrast, diffusion models, which are primarily used for image creation, take a more gradual approach by iteratively refining the distribution of pixels until the desired image is formed.
Frontier models are pushing the boundaries of what is possible. These large-scale systems can perform a wide variety of tasks and often surprise us with their capabilities. To ensure the safe and responsible development of these advanced AI programs, tech companies have formed the Frontier Model Forum, which aims to share knowledge, set safety standards, and promote understanding among stakeholders.
By understanding the fundamental concepts and latest developments in the field, we can better prepare ourselves for the future impact of AI. At least that's my hope.
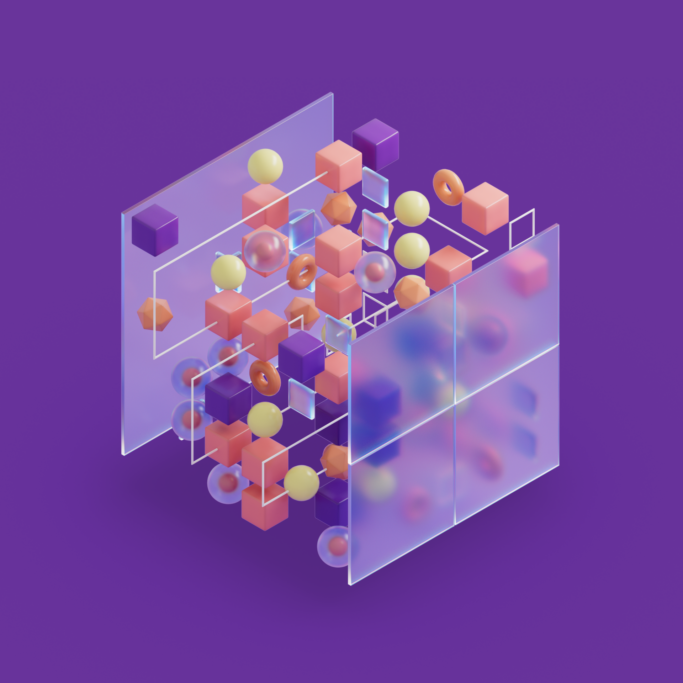